Autopilot for Elite Dangerous using OpenCV and thoughts on CV enabled bots in visual-to-keyboard loop
Ok, first let’s get one thing clear. This is an educational project for me learning some computer vision algorithms (I want to make an insect identification system to protect a bee hive, – but that is for much later article) and the game Elite Dangerous provided only interesting guinea pig here to test some principles. This was never intended as a game cheat/bot or anything like that, although in the last chapter I will give my thoughts on AI becoming a thing playing games using undetectable external “human loops” (e.g. looking at monitor and pushing keyboard) that no anti-cheat will ever catch, but that is way beyond my motivation as I personally like Elite as it is and definitely do not want to destroy its internal mechanics by creating a farming/trading bot this way. That is also the reason why code of my experiments is not disclosed. If I do not figure out some clever way how to share this without some script-kiddies turning this experiment into a cheat I never will share this code. If you are here looking for a game cheat, you will not get it. If you are here to learn how to program a cheat for yourself then kudos to you as I am trying to elaborate in the conclusion parts below, this is not really preventable. But it is your responsibility and try to think hard if your are not destroying the very game you like (but you might learn some skills for your carrier in the process).
So now that this is said, lets look at the interesting challenges of using computer vision (provided by OpenCV library) to analyze flight in Elite Dangerous.
Contents
Preamble. Why Elite Dangerous?
Simple, combination of two things, first I like this game and I am familiar with it and secondly this is a game that has a 3D rendered perspective GUI that shifts position, size and rotation during a flight via simulated inertial forces. This makes it a good experiments platform for trying to create for example algorithmes that a physical humanoid robot would face trying to drive a car. Comparing that to a flat 2D GUI, like some old games had, this introduces challenges that I wanted to focus one. E.g. not simply reading the specific instrument, but actually finding that instrument in real time in some 3D space and adjust the reading of that instrument via 3D perspective transformations. Have a look below on the difference between old 1991 game called “F29 Retaliator”, that GUI is simple to find and analyze, worst case you can write per-pixel classification for that. Nothing like Elite has or what you as human experiences when driving a car.
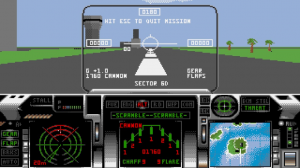
And even worse the Elite’s instruments are partly transparent, which makes it super hard for even the more advanced algorithms to work with because they can be literally blinded by in-game objects. E.g. the navigation target pointer appearing behind a sun, or even cockpit reflections when you are close to a sun literally destroy some classification algorithms (Keypoint family) ability to locate that instrument on the screen. Example below, in that situation nothing can read the top-left panel, not even humans.
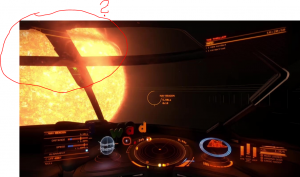
So despite all this, there is “something” possible and we will investigate this together below, just keep in mind all that we do here has a “Statistical” success rate. Meaning no classification presented here will work 100% and that is why creating a playing bot is also going to have a statistical results.
Part I. Investigation of basic “of the shelf” computer vision capabilities
As stated before, I wanted to learn CV (computer vision), and after some research I gravited to OpenCV (https://opencv.org/) and because I am lazy when prototyping, to the python wrapper around it (OpenCV-python-Tutorials-page). The python wrapper is using C++ library in the background so it is not such a bad performance as you would expect if this was all vanilla python. So you get the easy rapid prototyping with python CLI, but calling a much more powerful C++ in the background.
Secondly, I do not want to make this article to a detailed OpenCV tutorial, because OpenCV already has absolutely great tutorials, this will just skim the surface by showing main types of algorithms available, so that later we can check how I used them in Elite.
Now what are the basic classes of problems you can learn there:
I.I. HSV color filtering
This is not really any special algorithm, only a trick how to filter colors that really interest you to make your life easier later in analytics. Here the point is to convert from RGB (red-green-blue) to something called HSV (Hue-Saturation-Value).
With image/video in this color representation, it is simple to find those colors (in specific intensity!) that are interesting for you, for our example, I have helped my system here by turning the cockpit GUI into green and then simply filtering it in a series of try-and-error by playing with a few sliders as shown here below.

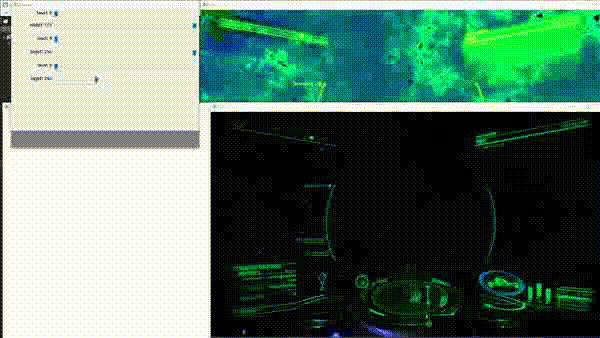
I.II. Template Matching
This is basically taking an small image as a target, and trying to find it in a larger image by the basic principle of moving the template image over the big image pixel by pixel and compute how much the color palette matches. This is computing intensive, and it can make a lot of false positives, especially if the template image is NOT present, because this algorithm is finding statistically best match, there will always be a best match, even if it is a nonsense.
Here is an example of how this can find a face on a photo, if you provide exactly the same fact as a template (courtesy of the above OpenCV tutorails):
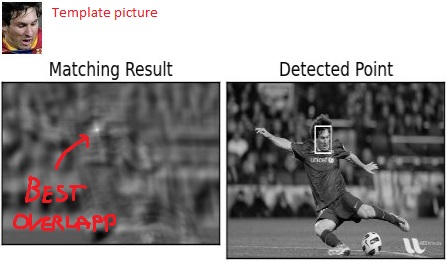
For Elite, this can be applied for finding the exact place where a template image is located, IF you know that the image is definitelly present, for example the NAV compass. The NAV compass has a specific shape that is not changing very much, so we can find it. To make it a bit simpler, we can limit the space in which the algorithm should look for it (this saves a lot of CPU power).
Additionally, if we find the NAV compass, we can cut it away and via HSV filtering, filter the blue dot to determine where it is (simple looking for white color in sectors). Here is result where on live feed we can find the NAV compass exact position, extract it, mask if via HSV and identify if where it is pointing (LEFT vs RIGHT, UP vs DOWN). This is a cool trick will be much useful later.
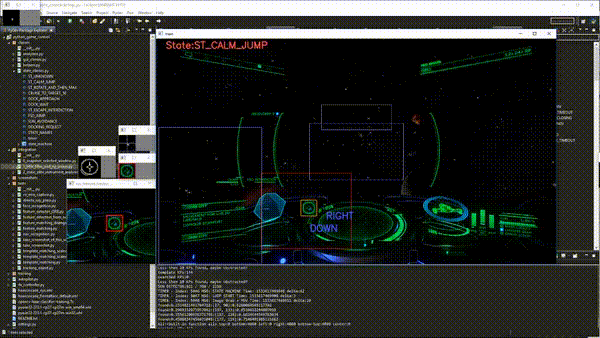
I.III. Keypoint Matching
The previous process has a biggest disadvantage that that it cannot detect or the presence or absence of the said template, this is where keypoint matching can help. This process takes a template, and tries to identify all the “corners” of the template. So this can detect a template image in bigger image, and also be rotation invariant (Ergo the picture can be rotated) and is partially robust against overshadowing.

For Elite dangerous, this is needed to actually identify different types of messages and objects presence on the screen, to give one of the more simple examples, Here is a video of these algorithms detecting nav beacon (the thing in the center where you want to go) and message to “throttle up” comparing the video to a template.

I.IV Haar Cascade
This is the only neural network image identification that you need to pre-train to find the object you are looking for, in my case, I was experimenting with this family of algorithms in the most complex task I could think of, and that is leaving the station. Which means finding the door out. Now this is much more complex that it sounds.
NOTE to help your own training: And it is extra hard for you as the creator because you need to feed the system with ideally thousands of pictures that contain the object you are looking for. The system will try to train itself based on these picture. And since OpenCV is an open-source with it’s typical “stability” of code and tutorials, I spend much time even learning the tools and picture format/types that it expected, so if you want to follow, I really expect you use this preparation scripts and process from mrnugged on github. This will save you hours!
The haar cascade trainer creates a set of features to look for with specific size and intensity (this is the training result) which can be appliead to the pictures. For simplest example (from OpenCV tutorials again), we have three basic features a – edge, b – line, c – four, the trainer will train for and it will apply them to picture as visible below:
Now in Elite, the results of a simple haar was not really great, but maybe because I only given the trainer several dozens of images and not tousands (come on, there is not enough elite dangerous pictures in google images like that). So I needed to combine it with something else to focus the haar more on the object itself, so I tried combining it with the template matching, together with some rotational and scaling brute-force variations, while haar cascade would then only say yes/no as a last clasification decision at the end.
The results are better than expected for a first try as I am definitely getting more than 50% correct identification, but not yet enough for a successful Elite control (since risk of collision-death here is high). Here is an example from prototype run trying to locate this template of a door exit:

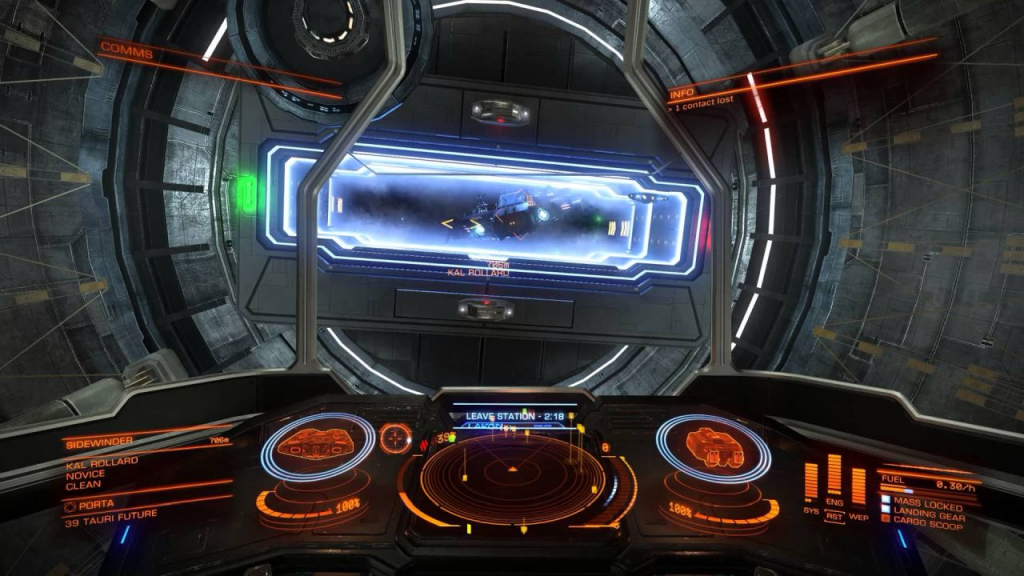
So here is a result of applying haar clasificator (simple training) together with template matching (rotational invariance and scale invariance via brute-force), consider the delays between images, that is how long the classifier is working, so it takes 3-4 seconds for each image. So this is great, but not applicable for live video or actually flying the Elite as a bot 🙁
I.V. Live tracking after object already identified in previous frames
This is a different family again, this time we are not identifying something, but keeping a track (e.g. position on screen) of an object that was previously identified. Again applying the OpenCV family of algorithms, this allows us to gain some framerate as you do not need to constantly identify objects (like in the above Haar classifier it took several seconds), you just keep track of object identified once.
There are several algorithms present, some are better but cannot handle scale changes or rotation, some are worse, but they can actually allow the object to “come closer” to the camera and scale the frame of reference. It takes time to experiment and think about your specific use case, but for our small Elite analysis, I am extending the task of finding the door from above, to simply tracking it.
So here is a simple example where I identified the doors manually with my mouse in the screenshot, then the code was tracking that door in a live video I made. And it was relatively successful (e.g. it tracks it, but imaging an autopilot going for the “center” of the box, on many times the box center is actually on a wall!! So not yet flight ready).

Part II. Creating a long-distance jumping and cruising autopilot
First of all, this is an autopilot, not an AI (in the true sense) and also not a full bot designed to farm or in any way cheat. Since it cannot really operate alone and get out of some dangerous situations alone. Here is a list of remaining things that would need to be solved for this to be considered a cheat, and some of these are much harder then you think:
- Survive interdiction, right now this autopilot if interdicted will try to return to path in full speed and engage FSD, but that is not really enough, no tactical use of countermeasures, and I tried creating a procedure to win the interdiction minigame, but that is not guaranteed at all.
- Get out of stations without dying or getting fined (which would in time make the station hostile)
- Understand the galaxy map to actually travel somewhere (prerequisite 1 to make a trading bot)
- Understand the communities market (text recognition that I haven’t covered yet, as prerequisite #2 to make a trading bot)
- Not hit dangerous stellar objects, I have written a procedure to avoid a sun (which was easy) but there are other nuance problems like hitting a black hole or a planet that is hidden in a shadow, or planets rings. These are hard to detect visually as they are not indicated in the hud in a simple way and are in many forms and shapes.
Sooooo … where can we apply this in our Elite Dangerous with these limits? Well simple, this can still be a relatively simple autopilot to help you make a few jumps to a destination and if combined with an in-game docking computer it can request docking at the very end so you end landed.
II.I – State machine, or “insert real AI here later”
This is the part where I will again emphasize that this is not an AI, because this autopilot is following a set of rules and states that I gave him, so it is a BOT, not an AI. It cannot learn from its mistakes or invent new states. It will be like an idiot continue following the states and conditions even if they were to lead him to a hazardous extraction site with cargo or full speed to neutron star. Nevertheless here are the states I created for a simple autopilot (with the limits mentioned above applied)

II.II – An example run of my simple Elite autopilot
After a long time I decided that we will do this part as a video. The below is a live demonstration of a full flight made of two FSD jumps, super-cruise and station approach.
Remaining limitations
To call this a proper autopilot, there is one last aspect missing… leaving a station. Despite some nice examples I have given above in the specific algorithms sections, this part of my autopilot remains very buggy, and therefore deadly. Classification by shape and color was successful maybe in 60%/70%, the remaining cases it either decided that another ships engine (because they often have the same color and from some angles also shape ) is the exit door or in some station types some building was a better candidate. Also for bigger ships (I am flying Imperial Cutter), there was just no way how to avoid collisions with other traffic inside the doors. If someone manages to solve this part, I would be happy if they share with me in the comments. For now my conclusion is that this is definitely also possible, but would require a much more robust classification and tracking system, but I assume if someone would really put together thousands of posivite/negative images of station doors, the neural net training would be able to crack this problem with higher success rate ….
Conclusion and thoughts on gaming
Well, my personal conclusion is that these were great skills that will later help me do my other home projects (I just really want that laser turret shooting wasps, but I need these algorithms for it to avoid shooting a bee .. e.g. “insects classification” AI training needed). However after the last 2 months the conclusion is that if there is a pattern in your favorite game that a relatively simple state machine can loop through, and doesn’t require very fast reactions (e.g. if you seen the video, this classification run maybe 8-11 FPS due high CPU load), there is a chance to build a grinding bot for it that will not be detectable by any anti-cheat system. Worst case you take another PC #2, point it’s camera at PC #1 running the game, and hook-up a keyboard interface so that PC #2 can somehow push buttons on PC #1 and you have essentially a “fake human” playing a game. This is what these advanced CV algorithms can do. It is not easy, it has only statistical success-rate, but it might be the next big AAA game economy killer if someone spends the large amount of hours coding+training something like that. …
…then again I do not like grinding in any game (…yeah, coming from a guy who owns Imperial Cutter in Elite, right…) as I do believe that is just lazyness from developers to avoid creating a proper story/tournament/sandbox system and if CV + AI technologies kill these games because it will become too easy to get an AI-bot to play the grinding parts instead of a player .. we might see a future where grinding games will not be developed anymore … who knows.
Feel free to let me know in the comments what you think about the AI vs grinding in games … and please avoid going into the traditional cheats/bots topic (e.g. CS:GO wall-hacks, or game money trainers) as that is really a completely different topic and actually cheats of the game itself via internal memory of a running game client is kind of lame and doesn’t deserve to be validated by even discussing it. Thanks!
I’am agree your opinion that bot kill the game, but I would find it useful a bot for long travel useless (20 minutes in buble for move for powerplay or move ship for engineer) and read book or see film 🙂
I think you can optimized of 30-50% calculation, with , gravidar, info target and ridodant alert in thread(with slow sleep) and add high sleep in thread not importants.
About “Live tracking after object already identified in previous frames”
In real life all tracking not are real time, obviusly if be tracking in real time is very huge calculation, 200ms sleep is sufficent for object speed at < 20m/s at range 500meters (relative velocity), in real life this is big problem for autopilot car, i think have 1 processor for warning state that check 10-20ms (warning state example object front and need break)
About the problems I can be there I want to help on one point:
5.Not hit dangerous stellar objects, I have written a procedure to avoid a sun (which was easy) but there are other nuance problems like hitting a black hole or a planet that is hidden in a shadow, or planets rings. These are hard to detect visually as they are not indicated in the hud in a simple way and are in many forms and shapes.
For not impact see "alert impact" in INFO in red is easy.
However in real life and in this case is impossibile training AI in "real life", AI is slow, I think that can programmer partially AI for management partialy calculation direction OR that create AI for learn by you, in this case 2.3 weeks(~200 hours) and be good what 2/3 of you.
Obsiusly if your language not permit sleep or multi threading I retire previus say.
Sorry my bad english.
Hi Shuttle Programmer,
Thanks for feedback, however I have already solved avoiding dangerous objects with a simple trick of calculating average brightness of center screen as a way to detect stars. If that happens I order the ship to to go 90 degree up for a few seconds and retry returning to the original course afterwards. Good enoug.
The bigger problem I am facing now is “un-docking computer” that can disconnect from a station and fly out the station main door. Finding the door and avoiding other ships is a major problem. If you find some algorithm for this, let me know. For now I am trying to train a deep learning AI to locate the door, then using motion tracker to fly to it. The biggest issue is that if the identification fails, e.g. you identify internal hangar object or building as the door … you are dead because motion tracker will not realize this mistake. So I need 99.9% reliable door identification from inside.
Peter
Firstly, once you have a median height established, the grill will always be at the same location, thought it will grow as you get near it. There are 2 lights, red and green on the centre line. Again centre these and use them as the rotation decider. The green side is also the safe side, albeit only safest not nec. free of objects. The UI rose on the dash shows all ships in your vicinity. Use this to check if the ship is right in front or/on the same level.
There is also another way and that is to perceive your ship as a known rectangular object, existing another rectangular object which rotates. Your job is to watch the rotation and rotate your own rectangle to match.
Heya Peter would you be interested in talking about this with us on Lave Radio?
Hi Cmdr,
Honestly this is such an early prototype that I do not want to go yet beyond this article with it. Maybe one day when I at least manage to safely finish the “un-docking autopilot” part that can get out of the station door without crashing my cutter ~40% of the time I might reconsider.
For now feel free to use any of the materials here in your site if you wish, or I can provide some raw footage.
Peter
I’d actually love to have you on the show to talk about the work you’ve done here even as it currently stands. We’d also talk about the moral implications which your obviously aware of and maybe things that Frontier can do to counter it.
I can obviously reference your work but it’s better out of the horses mouth as it were. For your things we’d keep it white hat and theoretical. You can get in touch with me better on our discord server which is linked to from our website
Cheers Cmdr Eid LeWeise
Hey Peter,
Cool project. I had kind of assumed that piloting would require a screen grab. A long processing time and then a response. But you’re hitting 10-14 FPS so that’s way cool.
I have an application for Open CV and Elite that you might be able to give some advice.
I need to capture the traffic report every half hour. Voice Attack can do most of that as a simple macro, and that’s fine, but the Traffic Report moves up and down in the menus.
So what I want is a routine that can click down, and scan for when the word “traffic” is highlighted.
It seems like it should be do-able but I’m trying to estimate how much of a learning curve I would have and how good Open CV is as an Optical Character Reader in the context of Elite Dangerous.
My email is “dna dot decay at g mail dot com”
Happy to chat in public if you prefer.
Fly safe CMDR o7
DNA-Decay
Hi DNA-Decay,
Ok, if you need to “read” the GUI, like missions list or market, I think you need to go for the same family of algorithms that are used in OpenCV for reading text off license plates and similar, and there is a lot of examples out there for that with OpenCV.
https://pbs.twimg.com/media/BzitiRkIYAEh1GE.jpg:large
I would personally recommend pyimagesearch.com and the tutorials that they sell* there for OpenCV + python, I haven’t done this part myself but I assume this license plates example should be easily searchable using google and then it is up to you to adopt for full screen reading all the menu texts.
My personal effort estimation is that this might be doable in a few days work, since in Elite you will always have the same font-type that might ease your detection and relatively the same color tone. And the performance for whole screen full of menus and text might be really bad, but I do not think you care about FPS when docked, right 🙂
Peter
*Yes, they sell OpenCV tutorials/examples book there, so this is not a sponsored link or anything, but I used them and I do appreciate someone putting this together in a readable way. Since I know first hand how hard it is to put together a learning material that makes sense end-to-end.
quote: “Since I know first hand how hard it is to put together a learning material that makes sense end-to-end.”
Your work here is much appreciated. It has entirely changed the nature of the debate about what is possible in Elite Dangerous. A tutorial like this so clearly laid out and step by step with actual solutions has silenced the doubters. And keeping the code under wraps is also appreciated.
Thanks for your leads, I’ll let you know how my project progesses once I get leave from work.
Great work, read it with great interest.
I’m on a similar project, currently doing some operations while docked.
My method for object recognition uses GDI functions, works not too well because of the blurry screen objects.
So I’m redesigning it using OpenCV.
I do the screen capturing with the GDI functions
GetWindowDC(NULL) GetWindowRect() BitBlt() and friends.
However, there is an issue wit the ED window:
when retrieving it with
GetWindowDC(hWnd)
the result is a black screen.
Works well with X-Plane or Notepad.
Currently i capture the entire screen with GetWindowDC(NULL)
Any hints?
Is there a OpenGV method to capture a desktop window?
Hi Xwg1,
First of all “may the force be with you!” … assuming you like X-Wings 😉
Secondly I do not think I can help you directly as looking at your function example, I believe you are using native C/C++ OpenCV, right ? I am using OpenCV library called from python, so my window capture is also written in python, using mostly mss library. Since this part of my code is not helping you with any bot farming, here is my script example for window capturing and converted to OpenCV compatible frame to be displayed in imgShow():
https://networkgeekstuff.com/article_upload/elite/snapshot_selected_window.txt
Just rename the file to PY extension to make it executable as python script, if will find a window in windows with specific name (using elite as example) and show a clone of that window in real time.
Thanks a lot, Peter!
Yep, I’m living in the C/C++ world, quite a while already, Unix, Linux and windows.
As I’m, collecting progamming languages 🙂 I very likely will dive into python to check what’s going on.
Does the python version of screen grabbing work with window being covered?
Have a nice day,
Wolf
PS: X-Plane is a flight simulator 🙂 – X-wings is new to me 🙂
Hi,
The python code actually doesn’t work when you cover the window with something else as it is essentially taking any pixels in the X,Y coordinates of the window found via windows window manager interface. But I never considered this a problem because for the game control (pushing directX keyboard inputs) you need the target window focused, which usually also means it will be on top of everything.
Peter
Ah, ok, so its the same as my c-version.
I’m just wondering whats the trick, because with other openGL apps like X-Plane etc. capturings works well with target not in foreground.
Anyway, thanks
Wolf
You can get the window handle of your choosing using standard windows findwindow functions, then get device context as normal. Should not matter then whether your window is in foreground or not.
I’m still discovering what is possible now with computer vision, using OpenCV seems very powerful yet their functions are well thought out. This post has been a real eye opener, nice one Peter! I’m also giving this a go in C/C++ following the methods of template matching outlined by Peter. Feel free to connect if you want to exchange notes
Where to connect to exchange notes?
I would love something like this. Set course for Colonia and click “go”. Then read a book or watch a movie and kinda baby sit to make sure no emergencies come up. There would be no real “cheat” involved in this. No systems scanned. No free money. Just jump, scoop, jump, hundreds of times. I mean, surely by the timeline of the game such technology would be invented for such long haul travel. And the annoyance of pointing the nose of the ship at a station and cruising having to sit the whole 10-15 minutes on the far ones babysitting it to make sure it doesn’t drift? How low-tech is this high tech world? LOL.
Hehe… that is exactly what I have here. The only problems are sometimes getting killed by black hole or running out of fuel. But I did managed to make a 500ly journey with this to satisfy one Engineers invite requirement. 🙂 Still things that need to be improved. And sorry but as mentioned in the article, giving you the code would mean spreading a cheat and me risking getting a ban 😉
But feel free to follow me with OpenCV, the learning experience is fun as well.
Hi there!
I’m on my way to Colonia once again. After a few dozen jumps I felt the need for an autopilot.. 🙂
A few minutes later Google brought me here. I’ve pulled the repo, mapped the keys, set the resolution to 1080p and started EDAutopilot. All green, both in the console and the log. Cool!
However, EDAutopilot was unable to find the jump target, even if I placed it just on 12 before starting the autopilot. It keeps flying loopings, searching for something, supercruise or not does not matter.
After reading this article and looking at the state machine flowchart I wonder, does EDAutopilot needs nav beacons to work? Because that obviously would be a show stopper on the way to Colonia. 😉
Or is it just the current Elite release which is not compatible?
To the fellow Elite pilots reading this, don’t get me wrong. I do not want to cheat or farm credits. I just like the idea of having a helpful computer at my side, for the 300 or so jumps on the way to Colonia.
Thanks,
Tom
Hi Tom,
LoL, this idea has taken a hold 🙂
FYI you first confused me with the repo and EDAutopilot as I didn’t released my code to avoid people using it for cheating since my code included already some automatic trading parts. So EDAutopilot is not my work, looking at the date tags, this article was published on August 2018 and first release of EDAutopilot I see in the repo from skai2, is on May 1019. I might ping the EDAutopilot author for thoughts but you are definitely contacting the wrong person here for problems with EDAutopilot. Different project, but similar indeed.
With regards,
Peter
Hi Peter,
Oops, wrong autopilot developer. 🙂
Your article is mentioned in the header of https://github.com/skai2/EDAutopilot/blob/master/dev_autopilot.py, so I assumed EDAutopilot was written by you. Well, if I’d read more carefully..
Anyway, I can’t convince you to release your autopilot?
Just remove the trading parts and anything else that generates credits in the game. A ‘supercruise assist’ and an ‘advanced docking computer’ are now officially in the game, so this part is covered by FD itself.
The one thing missing is a jump assist. During my last trip to Colonia, which in fact was just pressing ‘j’, waiting in the tunnel, honking, align the ship and then ‘j’ again for about five hours I had time to watch two movies while ‘playing’. Because of the movies I crashed into a yellow ball more than a dozen times. However, that’s no longer a problem in the current release, as there are unlimited heatsinks through synthesis.
On the other hand, isn’t that what we love about Elite? Not playing while we are playing? Surely this is some Zen in there.. 😉
Cheers,
Tom
Well, about the danger of stars, my stupid method at the beginning (as I was lazy writing star classification AI) that I tried as very safe settings for long travels, sacrificing speed was that whenever FSD jump ends, I rotated the ship 90 degrees up, full throttle for 15 seconds. Afterwards you are far enough from any “end of jump star” that the autopilot can simply rotate freely and FSD jump again. Later iterations simply stopped after each FSD, rotated to next hop and detected if the yellow circle was full or with dotted line (obscured by a star) and if former jumped from standstill (e.g. full throttle only after full FSD charge) or did the 15s up circle if obscured.
About the code release, I haven’t played this for a while and since ED changed the graphics a little since last yer (don’t remember the update number where the graphics lightning was changed) my autopilot has to be retrained completely to recognize the new color pallet properly. So right now it is very much useless without your understanding the code and retraining. Which is on the point that you can probably adjust EDAutopilot or write your own right now.
I moved now to andrupilot trying to pilot a quadcopter, not sure if I return to Elite (I have my A rated Cutter now, although trip to Colonia I never done, so maybe later).
Peter
Hi Peter,
back again after a long pause…
Watching your sample video I wonder what method u use tracking the navcpompass.
I’m using HoughCircles and it works well most if the time.
I’m aiming a ‘perfect’ method to readout navcompass indications; next I’m gonna try feature detection (SURF).
My final goal is to find reliable methods to read cockpit instruments, e.g. for an aircraft.
Wolf.
You are overdoing it with very complicated systems. Sometimes a simple approach is more powerful. I am simply doing a template mapping masking. E.g. in a given area of the screen I am looking at the exact pattern of the nav beacon. .. from that I know exactly where the beacon is and I extract it as a square picture.
Now on this picture of a beacon, I do a second masking to isolate the small blue dot and then I simply calculate if the blue dot is closer to TOP, BOTTOM, LEFT, RIGHT by simply counting the color average for the whole sector of the isolated picture and turn the ship in the same way.
Thanks to the optimized C library under OpenCV even counting all the pixels in small picture for knowing if there is more of blue color on left or right side of a square area is really fast, but also reliable.
In fact, this is just a part, it is necessary to filter out the extra circles, and they can really be on the mini-map in the form of stars and to the left in the form of a 3D target model.
The same point can merge with the background and quite often.
Thanks for your comment.
I’ve also implemented template matching with mask and yes, works well if no sun is above/ahead glaring the instruments/navcompass.
I’m using semi self learning for this, saving the images when below 0.995%.
I’m gettin lotta images this way :-), so I have to improve it.
And yes, getting the dot appears being a minror issue.
Wolf